The Power of AI Prompts: Top 5 Techniques with ChatGPT-4
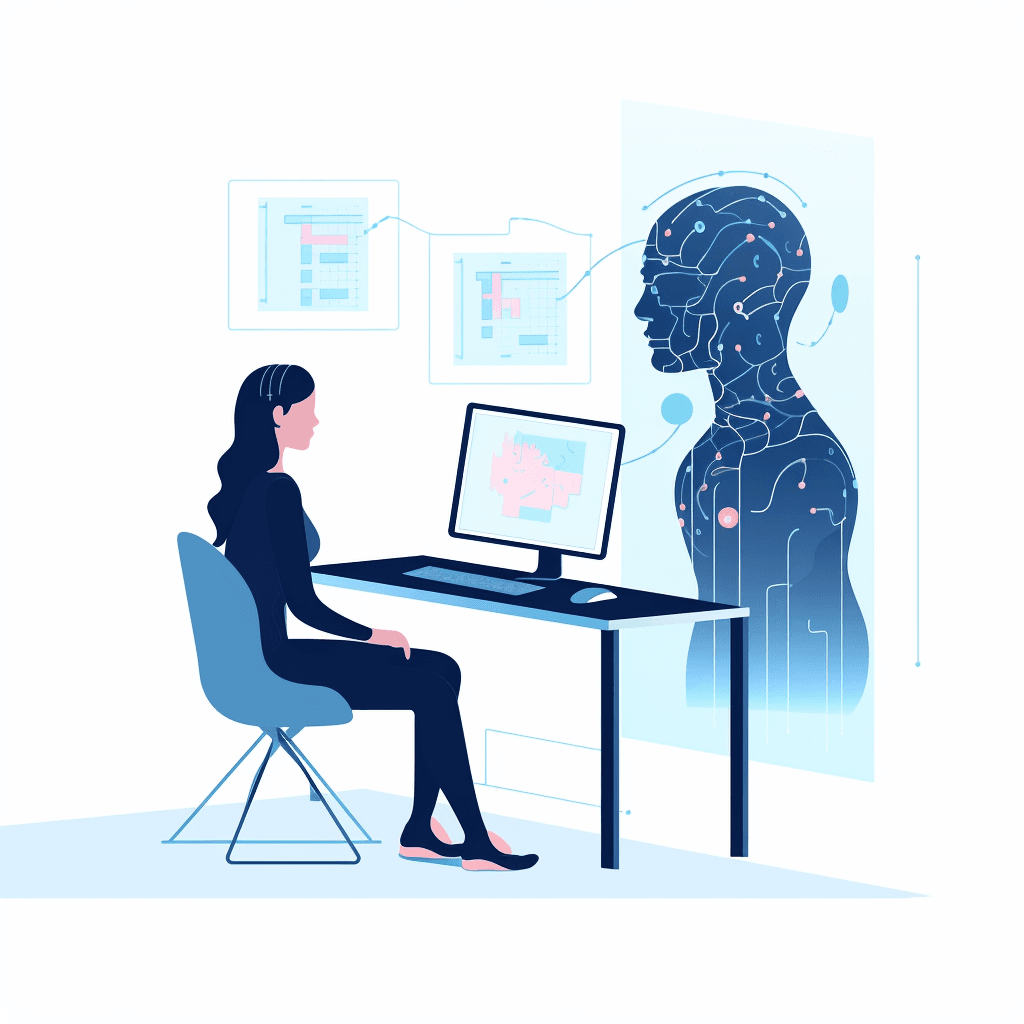
The Power of AI Prompts: Top 5 Techniques with ChatGPT-4
What if I told you that the key to unlocking the full potential of AI is all in the art of asking the right questions? Welcome to the fascinating world of prompting. In essence, prompting is how we communicate with large language models, like ChatGPT. It's a craft, and like any craft, it has its tricks and techniques. In this article, we're about to dive into the top 5 professional prompting techniques, techniques that will allow you to guide the AI, streamline its output, and get the responses you're truly looking for. Get ready to take your AI communication skills to the next level!
1-SHOT LEARNING
Let’s first talk about SHOT LEARNING. It's all about EXAMPLES we give to Language Learning Models, like our buddy ChatGPT, to shape its responses. We've got a few types in the mix: zero-shot, one-shot, and few-shot learning.
ZERO-SHOT LEARNING
The model can spit out some pretty solid answers without a single example to work off. Let's check out an instance:
Prompt: What are the main themes explored in the novel “To Kill a Mockingbird” by Harper Lee?
ChatGpt4's Response: “To Kill a Mockingbird” by Harper Lee takes a deep dive into themes like racial injustice, moral growth, social inequality, and the essential need for compassion and understanding.
ONE-SHOT LEARNING
We're talking about giving ChatGPT a single example to build its response.
Example: > Prompt: Example: What type of animal is a dog? Response: Mammal. Now tell me what the type of animal a snake is?
ChatGpt4's Response: A snake? That's a reptile.
FEW-SHOT LEARNING
This time, ChatGPT gets a handful of examples to understand the task.
Example: > Prompt: Examples: Apple: fruit Carrot: vegetable Salmon: fish So, how would you classify a “walnut”?
ChatGpt4's Response: Going by the examples, a walnut gets the label of a nut.
2-CHAIN-OF-THOUGHT (CoT)
Let’s move on to focus on a term you might not have come across before: CHAIN-OF-THOUGHT (CoT). We're talking about guiding Language Learning Models, or LLMs (think ChatGPT), through logical steps to get to the end goal.
ZERO-SHOT CoT
This means asking the LLM to simply "think or reason step by step" to work out a solution.
Prompt: Mary and John are battling it out in a game. Mary scores 3 points, then John racks up 5. Mary's back in with another 4 points and John adds 3 more to his tally. So, who's got more points?
ChatGpt4's Response: Looks like Mary's in the lead.
CHAIN-OF-THOUGHT with FEW-SHOT
Toss in a couple of examples, and it nudges the LLM towards using this approach and reaching the right answer.
Prompt: Mary and John are in a game face-off. Mary scores 3 points, then John steps up with 5. Mary counters with another 4 points and John answers with 3 more. Who's got the upper hand? Let's break it down step by step.
ChatGpt4's Response: First up, Mary bags 3 points and John nets 5, giving John a 2-point lead. Then, Mary adds 4 points to her score, making a total of 7 points. John follows with 3 more points, bringing his score to 8. So, the winner is...John!
3-META-PROMPTING
There’s an innovative approach to using AI, a technique known as META-PROMPTING. This one is a cool technique because it turns an LLM, a large language model like ChatGPT, into a creative teammate in our problem-solving journey, rather than just a tool that does what it's told.
Example 1
Prompt: Fire off three questions about the future of AI.
ChatGpt4's Response: What are the key ethical concerns we need to tackle as AI tech evolves and becomes even more ingrained in everyday life? How might AI tech shake up the job market and employment in the coming decade? How could the strides in AI tech possibly reshape human social interactions and relationships in the future?
Example 2
Prompt: Dream up a prompt for a story set in a dystopian future.
ChatGpt4's Response: In a bleak future where tech has outstripped human intelligence, chart the journey of a band of rebels who stumble upon an old-world manual on human creativity and emotion. How do they harness this knowledge to spark a revolution?
4-PROMPT-TRAINING SIMILARITY
The basic idea here is to line up your prompt with the type of data the LLM was trained on for that specific task. You’re not only aligning the content of your context with the desired response but also its structure. It's like matching your language to the language of the training data to get the best results.
Example
Prompt: I'm looking for an academic rundown of our current understanding in the field of Quantum Cryptography. Specifically, I'm interested in Quantum Key Distribution (QKD) and its significance, as introduced by Bennett and Brassard in their groundbreaking 1984 paper (Bennett & Brassard, 1984).
ChatGpt4's Response: Quantum Cryptography, a growing sector in the world of infosec, uses the principles of quantum mechanics to protect communications. One standout aspect of this field is Quantum Key Distribution (QKD), a fresh take on key exchange brought to the table by Charles H. Bennett and Gilles Brassard in 1984 (Bennett & Brassard, 1984).
5-BIAS AWARENESS TECHNIQUE
Now, this is all about getting large language models (LLMs), like ChatGPT, to check themselves for biases in their responses. It's kind of like putting a mirror up to the AI to get it to reflect on what it's saying.
Prompt: I want you to talk about the pros and cons of electric vehicles. Be aware of any biases in your response, offer different viewpoints on this topic, and evaluate your final response for any bias.
ChatGpt4's Response: When it comes to electric vehicles (EVs), there are a few standout benefits. They can help slash greenhouse gas emissions, reduce reliance on fossil fuels, and offer a less noisy, smoother driving experience. But, it's crucial to look at the other side of the coin too. There are challenges that come with EVs. Battery production and disposal can impact the environment, EVs can be pricey upfront, and the infrastructure for charging is still being built in many places. Looking back at this response, it seems fairly balanced, recognizing both the highs and lows of EVs. Still, the wording might come across as leaning a bit toward EVs, given the more upbeat language used to describe their advantages. In the future, I'll aim for an equal focus and neutral language when talking about both the benefits and drawbacks.
And that's a wrap on our deep dive into the world of AI prompting techniques. From SHOT LEARNING, helping the AI learn by example, to CHAIN-OF-THOUGHT prompting, guiding the model through logical steps, to META PROMPTING, stirring the creative juices of the AI. We then looked at the PROMPT-TRAINING SIMILARITY, which stresses the importance of aligning your prompts with the model's training data, and lastly, the BIAS AWARENESS technique, promoting self-awareness and introspection within the AI model. These techniques offer a robust toolkit to elevate your interaction with AI, ensuring you receive precise, unbiased, and innovative responses. So, next time you chat with an AI, remember these techniques and see how they can transform your experience!
Ali Razavi : LLM Researcher | Innovative Prompt Engineer | Developer of the First Architecture for LLM Agents | Author | Creator of the Internet's Largest Collection of Prompt Engineering Techniques (Prompting1.com/guide)